With AI upon us, companies have recently been turning their attention to “synthetic” user testing — AI-driven research that replaces UX research. There, questions are answered by AI-generated “customers,” human tasks “performed” by AI agents.
However, it’s not just for desk research or discovery that AI is used for; it’s an actual usability testing with “AI personas” that mimic human behavior of actual customers within the actual product. It’s like UX research, just… well, without the users.
If this sounds worrying, confusing, and outlandish, it is — but this doesn’t stop companies from adopting AI “research” to drive business decisions. Although, unsurprisingly, the undertaking can be dangerous, risky, and expensive and usually diminishes user value.
This article is part of our ongoing series on UX. You can find more details on design patterns and UX strategy in Smart Interface Design Patterns 🍣 — with live UX training coming up soon. Free preview.
Fast, Cheap, Easy… And Imaginary
Erika Hall famously noted that “design is only as ‘human-centered’ as the business model allows.” If a company is heavily driven by hunches, assumptions, and strong opinions, there will be little to no interest in properly-done UX research in the first place.
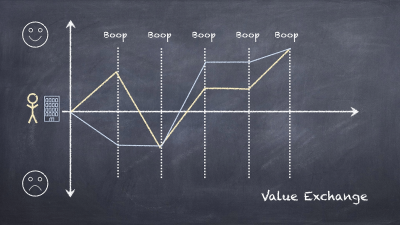
But unlike UX research, AI research (conveniently called synthetic testing) is fast, cheap, and easy to re-run. It doesn’t raise uncomfortable questions, and it doesn’t flag wrong assumptions. It doesn’t require user recruitment, much time, or long-winded debates.
And: it can manage thousands of AI personas at once. By studying AI-generated output, we can discover common journeys, navigation patterns, and common expectations. We can anticipate how people behave and what they would do.
Well, that’s the big promise. And that’s where we start running into big problems.
LLMs Are People Pleasers
Good UX research has roots in what actually happened, not what might have happened or what might happen in the future.
By nature, LLMs are trained to provide the most “plausible” or most likely output based on patterns captured in its training data. These patterns, however, emerge from expected behaviors by statistically “average” profiles extracted from content on the web. But these people don’t exist, they never have.
By default, user segments are not scoped and not curated. They don’t represent the customer base of any product. So to be useful, we must eloquently prompt AI by explaining who users are, what they do, and how they behave. Otherwise, the output won’t match user needs and won’t apply to our users.
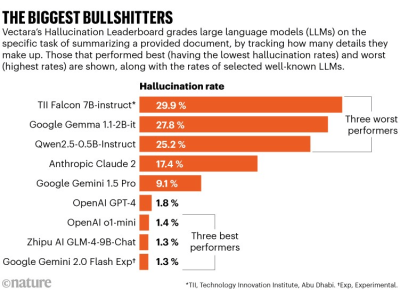
When “producing” user insights, LLMs can’t generate unexpected things beyond what we’re already asking about.
In comparison, researchers are only able to define what’s relevant as the process unfolds. In actual user testing, insights can help shift priorities or radically reimagine the problem we’re trying to solve, as well as potential business outcomes.
Real insights come from unexpected behavior, from reading behavioral clues and emotions, from observing a person doing the opposite of what they said. We can’t replicate it with LLMs.
AI User Research Isn’t “Better Than Nothing”
Pavel Samsonov articulates that things that sound like customers might say them are worthless. But things that customers actually have said, done, or experienced carry inherent value (although they could be exaggerated). We just need to interpret them correctly.
AI user research isn’t “better than nothing” or “more effective.” It creates an illusion of customer experiences that never happened and are at best good guesses but at worst misleading and non-applicable. Relying on AI-generated “insights” alone isn’t much different than reading tea leaves.
The Cost Of Mechanical Decisions
We often hear about the breakthrough of automation and knowledge generation with AI. Yet we often forget that automation often comes at a cost: the cost of mechanical decisions that are typically indiscriminate, favor uniformity, and erode quality.
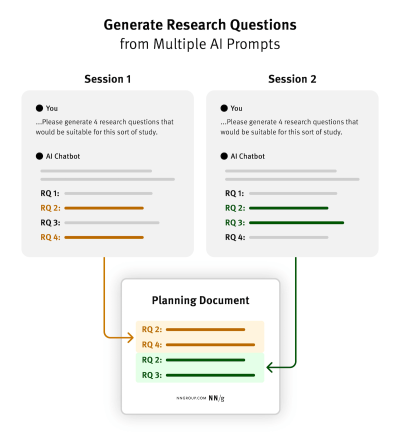
As Maria Rosala and Kate Moran write, the problem with AI research is that it most certainly will be misrepresentative, and without real research, you won’t catch and correct those inaccuracies. Making decisions without talking to real customers is dangerous, harmful, and expensive.
Beyond that, synthetic testing assumes that people fit in well-defined boxes, which is rarely true. Human behavior is shaped by our experiences, situations, habits that can’t be replicated by text generation alone. AI strengthens biases, supports hunches, and amplifies stereotypes.
Triangulate Insights Instead Of Verifying Them
Of course AI can provide useful starting points to explore early in the process. But inherently it also invites false impressions and unverified conclusions — presented with an incredible level of confidence and certainty.
Starting with human research conducted with real customers using a real product is just much more reliable. After doing so, we can still apply AI to see if we perhaps missed something critical in user interviews. AI can enhance but not replace UX research.
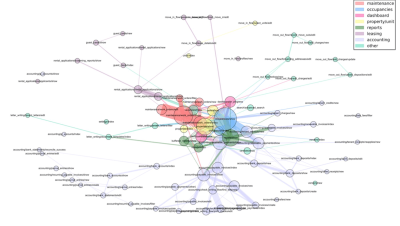
Also, when we do use AI for desk research, it can be tempting to try to “validate” AI “insights” with actual user testing. However, once we plant a seed of insight in our head, it’s easy to recognize its signs everywhere — even if it really isn’t there.
Instead, we study actual customers, then triangulate data: track clusters or most heavily trafficked parts of the product. It might be that analytics and AI desk research confirm your hypothesis. That would give you a much stronger standing to move forward in the process.
Wrapping Up
I might sound like a broken record, but I keep wondering why we feel the urgency to replace UX work with automated AI tools. Good design requires a good amount of critical thinking, observation, and planning.
To me personally, cleaning up after AI-generated output takes way more time than doing the actual work. There is an incredible value in talking to people who actually use your product.
I would always choose one day with a real customer instead of one hour with 1,000 synthetic users pretending to be humans.
Useful Resources
New: How To Measure UX And Design Impact
Meet Measure UX & Design Impact (8h), a new practical guide for designers and UX leads to measure and show your UX impact on business. Use the code 🎟 IMPACT
to save 20% off today. Jump to the details.
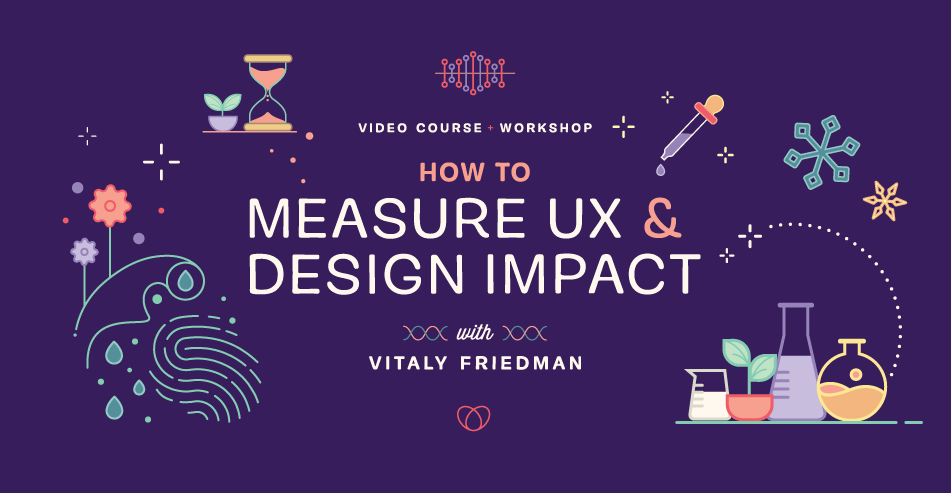

(cm)